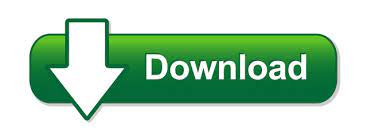
These methods should be used sparingly, because one can never be sure that an imputed correlation is appropriate (correlations between baseline and final values will, for example, decrease with increasing time between baseline and final measurements, as well as depending on the outcomes and characteristics of the participants). Note that the methods in (2) are applicable both to correlation coefficients obtained using (1) and to correlation coefficients obtained in other ways (for example, by reasoned argument).
#Error weighted standard deviation how to#
Here we describe (1) how to calculate the correlation coefficient from a study that is reported in considerable detail and (2) how to impute a change-from-baseline standard deviation in another study, making use of an imputed correlation coefficient. A typically unreported number known as the correlation coefficient describes how similar the baseline and final measurements were across participants. The following alternative technique may be used for imputing missing standard deviations for changes from baseline (Follmann 1992, Abrams 2005). However, the appropriateness of using a standard deviation from another study relies on whether the studies used the same measurement scale, had the same degree of measurement error and had the same time periods (between baseline and final value measurement). When change-from-baseline standard deviations for the same outcome measure are available from other studies in the review, it may be reasonable to use these in place of the missing standard deviations. When there is not enough information available to calculate the standard deviations for the changes, they can be imputed. confidence intervals, standard errors, t values, P values, F values) then the techniques described in Chapter 7 (Section 7.7.3) may be used. If statistical analyses comparing the changes themselves are presented (e.g. Some other information in a paper may help us determine the standard deviation of the changes. We cannot know whether the changes were very consistent or very variable. However, the information in this table does not allow us to calculate the standard deviation of the changes. the integral.Note that the mean change in each group can always be obtained by subtracting the final mean from the baseline mean even if it is not presented explicitly. Thus, for a continuous random variable the expected value is the limit of the weighted sum, i.e. Since probability can never be negative (although it can be zero), one can intuitively understand this as the area under the curve of the graph of the values of a random variable multiplied by the probability of that value. The weights used in computing this average are the probabilities in the case of a discrete random variable (that is, a random variable that can only take on a finite number of values, such as a roll of a pair of dice), or the values of a probability density function in the case of a continuous random variable (that is, a random variable that can assume a theoretically infinite number of values, such as the height of a person).įrom a rigorous theoretical standpoint, the expected value of a continuous variable is the integral of the random variable with respect to its probability measure. In other words, each possible value the random variable can assume is multiplied by its assigned weight, and the resulting products are then added together to find the expected value. More formally, the expected value is a weighted average of all possible values. In probability theory, the expected value refers, intuitively, to the value of a random variable one would “expect” to find if one could repeat the random variable process an infinite number of times and take the average of the values obtained.
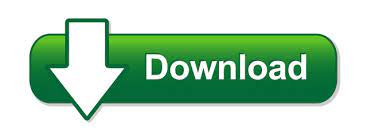